Welcome to “Why You Should Join,” a monthly newsletter highlighting early-stage startups on track to becoming generational companies.
As engineers ourselves, we know how difficult it is to pick the right startup to join. Doing your own analysis for every TechCrunch article, recruiter InMail, or VC tweet would be impossible. Let us help you out instead :)
On the first Monday of each month, we cut through the noise by recommending one startup based on thorough research, original analysis, and inside information from partnered venture firms. We go deeper than any source out there to help ambitious new grads, FAANG veterans, and experienced operators find the company to join. Sound interesting? Join the family and subscribe here:
Why You Should Join Hebbia
(Click the link to read online).
In the early 1220s, English philosopher and theologian Robert Grosseteste faced a rare but serious problem: he had too many books.
As chancellor of Oxford University, Robert would often lecture on theological topics like the Book of Genesis, the Psalter, and the Pauline epistles. Preparing these lectures often took a long time: researching a new topic usually meant going through his entire library to find the right thoughts, passages, and quotes.
Robert had too many books.
Robert had too much knowledge.
To address his problem, Robert began recording where specific topics were mentioned in a separate manuscript. Titled Tabula Distinctionum, this new document was split into nine top-level themes and 440 sub-themes. Each topic in Tabula had a unique symbol and list of references: by going through the sources attached to a topic of interest, Robert could pull the relevant book, turn to the relevant page, and find the relevant symbol (usually drawn in the margin) to pick out the right quote or paragraph.
It was a complicated system, but it certainly beat spending hours trying to find something he’d already read.
Robert had created the world’s first index.
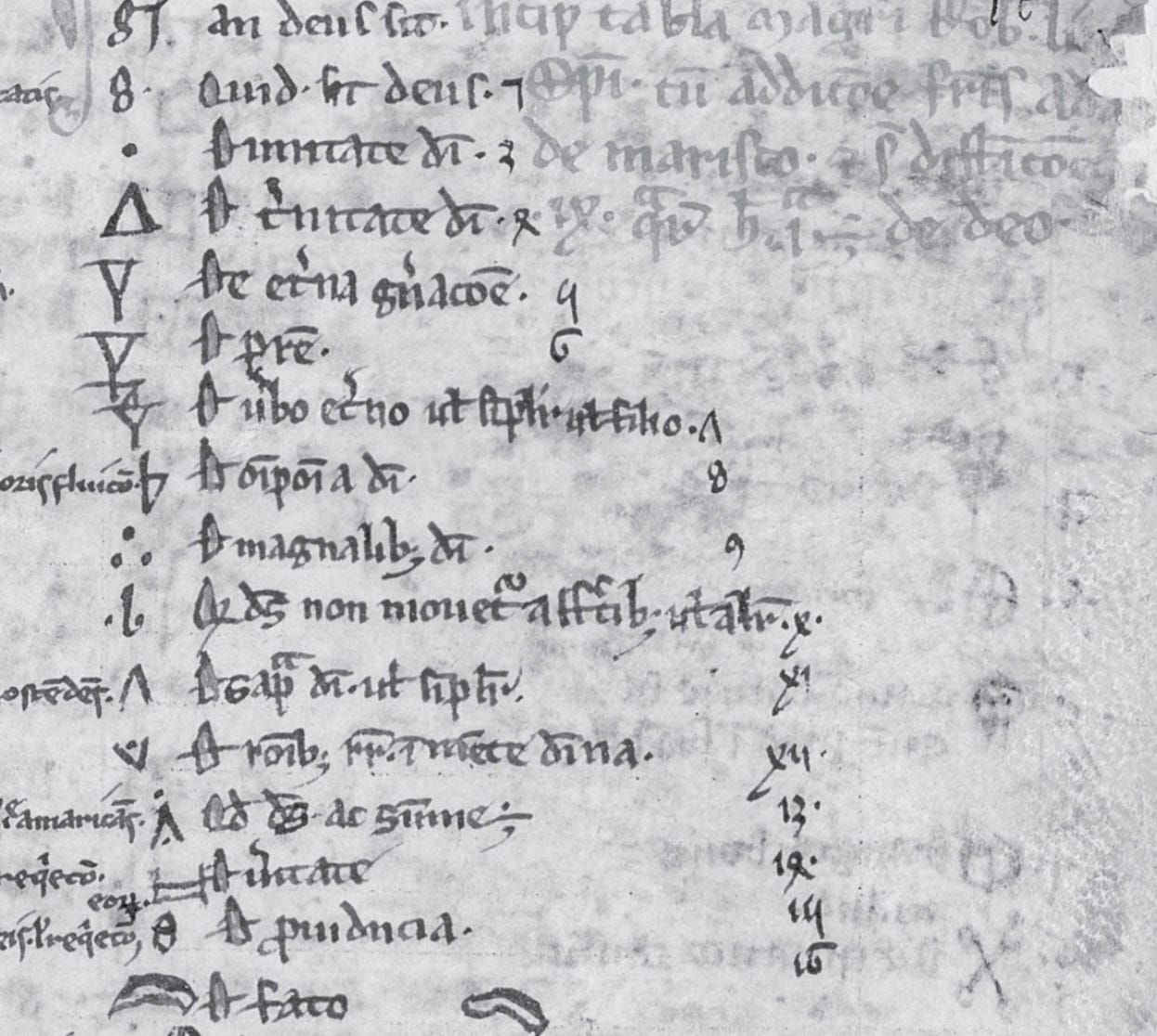
Everyone knows about the incredible progress in our ability to create and store information. Most are familiar with how over the past 3000 years, we’ve gone from songs to tablets, tablets to books, and books to semiconductors.
Less advertised has been the incredible progress in our ability to search that information. Robert’s index was a landmark in the history of information retrieval, but following it were tables of contents, keyword indices, and the Dewey Decimal System. The rise of digital information brought with it tools like SQL, Excel, and Command+F, and the internet gave us PageRank.
Information is only as useful as it is accessible.
As the world’s learned to generate more and more of it, we’ve had to develop ever more clever and efficient ways of searching through it all. Some of the world’s most valuable companies, the defining ones of the information era, were innovators in this regard.
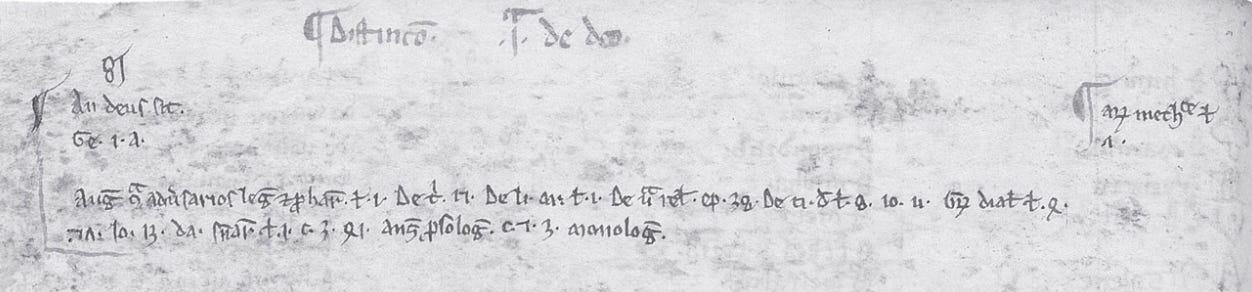
The thing is, we’re once again approaching the limits of our current capabilities.
Information, particularly unstructured information, is exploding.
Over the next 5 years, the world’s total data is set to compound at 21.2% annually. By 2026, we’ll have over 221,000 exabytes (221 billion terabytes) to deal with - more than two and half times what we have today. Worse, 90% of that data (80% for enterprises) is going to be unstructured - stored in the form of PDFs, emails, Word documents, Slack messages, and file scans, all things we don’t have great ways of searching through. The rise of generative systems like ChatGPT will only make the problem worse: we’re approaching an inflection in the amount of unstructured data we’ll all have to make sense of.
Like in the past, a new era of information creation will require new ways of searching through and understanding it. A table of contents and Control+F might have done it for us in the past, but we’ll almost certainly need a better tool for the future.
That’s exactly what Hebbia is building.
Background
Hebbia is building neural search for knowledge workers. Instead of relying on Command+F to search through large numbers of documents, people like investors, lawyers, and consultants can simply ask Hebbia questions in natural language: Hebbia’s engine will then use artificial intelligence to automatically identify, summarize, and cite the most relevant sources.
For example, instead of Command F-ing every PDF, Word Doc, or Excel file for “intervening” or “earthly affairs”, Robert could simply ask “What causes God to intervene in earthly affairs?” Hebbia would then pull, summarize, and cite the sentences and paragraphs best answering that question — even if the words “intervening” or “earthly affairs” never show up.
It’s a cheat code for any job where you read a lot of documents.
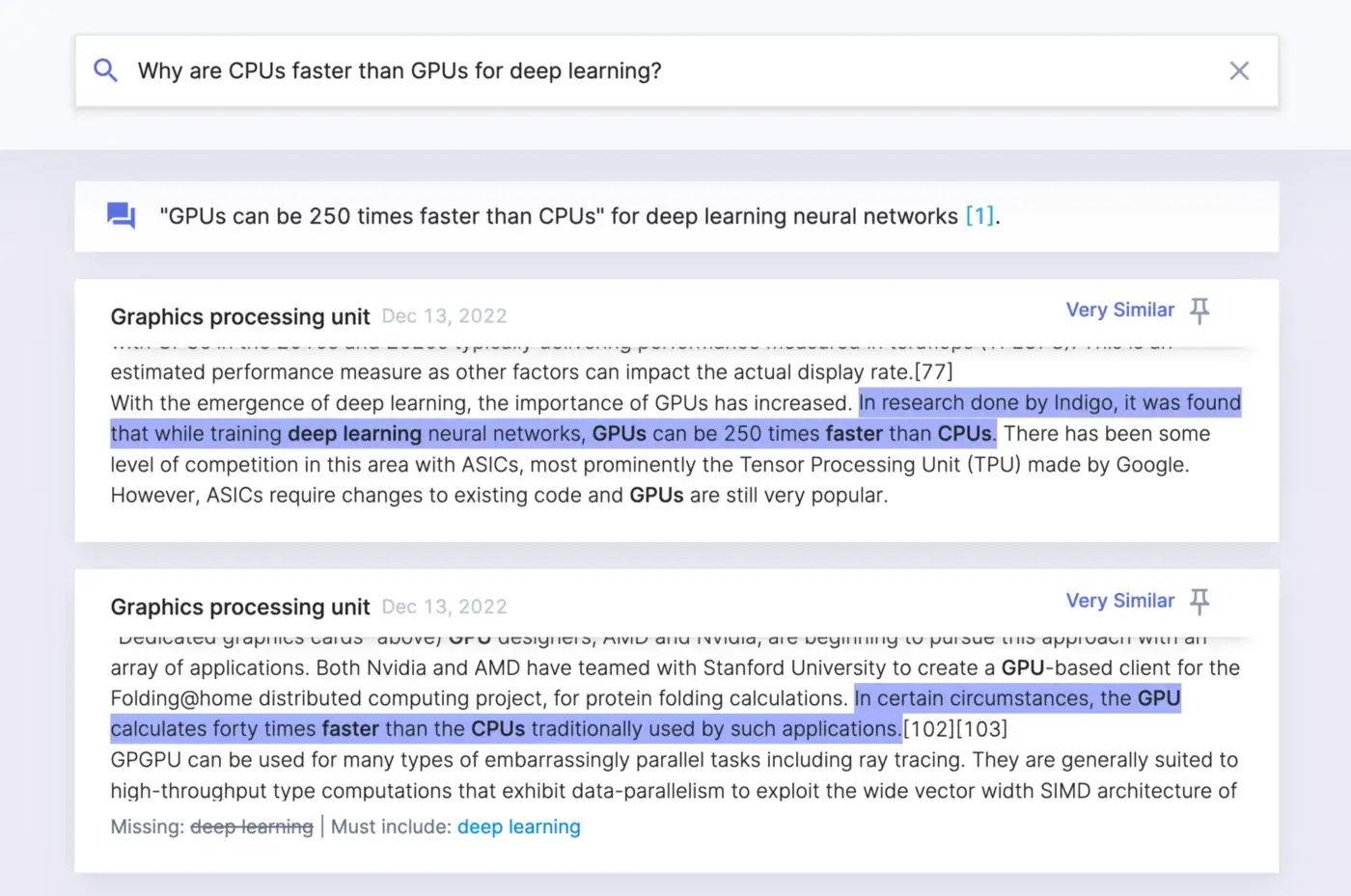
This fundamentally new approach to search creates value for users in 2 ways:
More accurate search. By considering the intent of your query, Hebbia prevents you from worrying about synonyms or phrasing like you would with keyword search: sources are ordered by how well they answer your question, not how much overlap there is between the hit and your input. Hebbia can find sources and facts you might have missed with just Command+F.
More efficient search. Hebbia’s MIME type-agnostic engine searches across all documents (PDFs, Excel files, Word documents, emails) at once. It automatically orders results using query intent and context so you don’t have to skip through each result like in Command+F. It even summarizes the results for you using LLMs, giving you an automatic first pass over everything.
Hebbia is offered as an end-to-end managed service. After uploading documents for the system to index, users can log into Hebbia’s web interface to run queries and answer questions.
The product functions as a force multiplier for highly paid knowledge workers: think private equity analysts diligencing hundred-million dollar deals, management consultants advising billion-dollar companies, or lawyers breaking down the 4155-page 1.7 trillion dollar 2023 Omnibus Appropriations bill.
It’s a premium product with premium pricing: Hebbia’s rates aren’t public, but they’re similar to what organizations might pay for a Bloomberg Terminal, Carta, or Pitchbook subscription.
Hebbia launched in 2021 with the initial goal of selling into top private equity firms doing diligence on multi-million dollar deals. They’ve been incredibly successful: in less than a year, Hebbia’s been deployed at 9 of the country’s 10 largest megafunds.
Since then, they’ve quickly begun expanding into other sectors of knowledge workers. Hebbia is currently used at three of the world’s largest consulting firms, a few government agencies, and several law firms.
The company was founded in 2020 by George Sivulka, a PhD dropout from Stanford’s Computational Neuroscience program. Prior to Hebbia, George had cold-called his way into a high school internship with NASA, finished a Stanford maths degree in under two and a half years, and climbed Mount Everest. He’s cited on the Wikipedia page for slow neutron-capture processes and was the first ever person to record the action potentials of five different species of Micronesian corals.
Most impressively though, he responded within 4 minutes when we cold emailed him to ask for an interview (at 2:30AM on a Friday night, no less).
We were impressed, and you should be too.
Investors certainly were. Hebbia’s impressive technology and traction got them a 30 million dollar Series A from Index Ventures in September of 2022. Before that, they raised a 1.1 million dollar pre-seed round led by Floodgate. Other investors include Radical Ventures, Human Capital, Peter Thiel, Jerry Yang (Yahoo), Alexandr Wang (Scale), Henrique Dubugras (Brex), Naval Ravikant (AngelList), Kevin Hartz (Eventbrite), Cory Levy (After School), and Ram Shriram (Google).
Hebbia’s excellent product, strong traction, and stacked team make them an exceptional startup. But are they a category-defining business?
We think they have the potential to be.
In this piece, we’ll dig deeper to see why this is the case. We’ll look at how they’re targeting a massive, globally important market. We’ll look at how they’re well-positioned to become a leader in that market. We’ll look at how they stack up against the competition, and why their team is perfect to execute on this opportunity. And we’ll do it all as thoroughly as we can.
Ready? Let’s begin.
Opportunity
We’ll begin our analysis of Hebbia like we do for all our companies, from a first principle:
Massive companies lead massive, growing markets.
Many companies with clear product-market fit don’t become truly massive because they don’t meet this condition. Thus, we must establish two things:
Hebbia operates in a massive, growing market.
Hebbia will become a leader in that market.
A Massive, Growing Market
Hebbia makes money by selling their software to anyone who regularly reads through and analyzes large amounts of information as a part of their job. Generally labeled “knowledge workers,” these include asset managers who read through large numbers of financial documents, lawyers who read through large numbers of contracts, and consultants who read through large amounts of market research. They also include any manager whose job involves reading through and making decisions based on their company’s internal collection of reports, contracts, and government filings.
While it’s hard to put an exact number on the size of this market, we think it’s pretty big for the following reasons:
There are lots of knowledge workers. Consider what the Bureau of Labor Statistics tells us about a few of the categories mentioned above:
There are 373,800 Financial Analysts in the country. These people guide individuals and businesses on investment decisions - think investment bankers, private equity investors, traders, and hedge fund employees. They have to read tons of documents to help analyze the performance of stocks, bonds, other investments. A better search tool would speed up their research significantly.
There are 730,800 Financial Managers in the country. These people manage the financial health of their organizations - think CFOs and the finance teams they lead. They have to read tons of documents to prepare their own financial reports and develop plans for their organization’s financial goals. A better search tool would speed up their research significantly.
There are 833,100 Lawyers in the country. They have to read tons of laws, contracts, and court rulings to help advise their clients on legal matters. A better search tool would speed up their workflows significantly.
There are 950,600 Management Analysts in the country. Often known as “management consultants,” they read through tons of market research, case studies, and internal documents to help advise clients. A better search tool would speed up their research significantly.
Knowledge workers are highly paid. Considering just the occupations mentioned above:
Financial analysts earn a median wage of $95,570 per year.
Financial managers earn a median wage of $131,710 per year.
Lawyers earn a median wage of $127,990 per year.
Management analysts earn a median wage of $93,000 per year.
At the high-end of the market (where Hebbia has focused first), knowledge workers can earn many multiples of this. These workers are highly leveraged in their time and effort: their business decisions command orders of magnitude more capital than what they’re already paid. Empowering a research team analyzing a 100 million dollar acquisition to move 10% faster is worth paying for - tens of millions of dollars are at stake with regards to corporate strategy and financial risk.
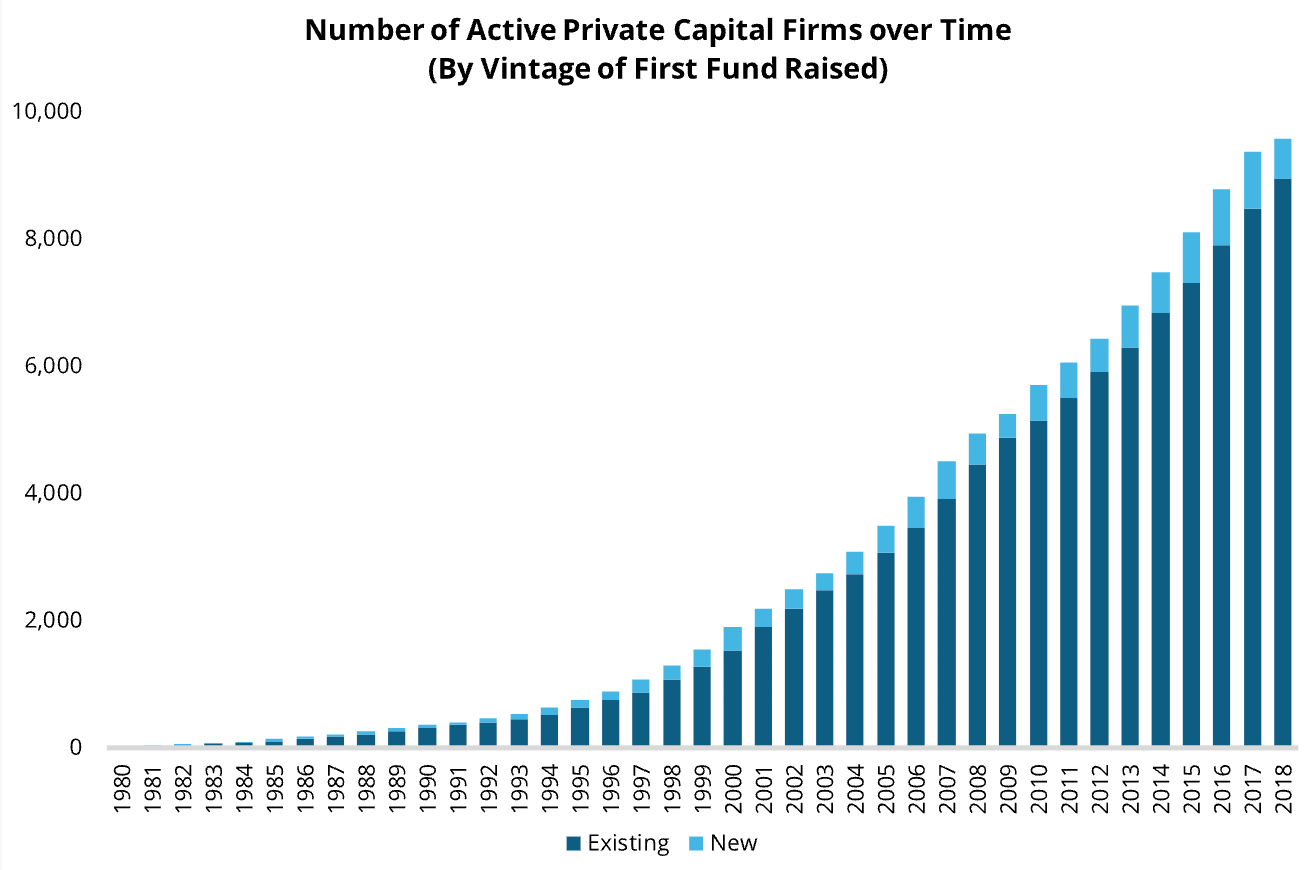
We also think the market is set to grow. Demand for tools like Hebbia will increase in the coming years for a few reasons:
There will be more knowledge workers. Over the next 10 years, the number of American financial analysts is set to grow 9%, about double the growth of the average occupation. In that same timeframe, the number of financial managers is set to grow 17%, over triple the growth of the average occupation. The number of lawyers is set to grow 10%, and the number of management analysts set to grow 11%, both over double the growth of the average occupation. More users means more demand for these tools.
Knowledge workers will have more data. By 2026, the world will have over 221 billion terabytes of information to deal with. Over 90% of it will be unstructured, meaning we don’t have great ways of searching through and analyzing it. Generative AI, which makes it easier to write and produce unstructured data, will only accelerate this trend. There aren’t enough human eyes to read and understand all of that information output; as the amount of data grows, we’ll need AI to help us understand it. More information means more demand for these tools.
Knowledge workers will make more money. Global mergers and acquisitions volume topped 5.8 trillion in 2021, up 64% from the year prior and an all-time high. The market for legal services grew 14% in 2021 and 5% in 2022. The global market for management consulting services is set to grow at a 8% each year between 2021 and 2028 to reach 236.4 billion, up from 160 billion in 2020. More revenue means more budget for these tools.
It’s worth capping this section by considering the broader opportunity at play here, something which can’t be captured in simple charts or numbers.
45 years ago, everyone managed and analyzed structured information by hand. That changed with the rise of spreadsheets: 1979’s Visicalc, 1983’s Lotus, and 1987’s Excel each revolutionized how we interact with and understand structured data. Today, tools like Airtable and Snowflake are still pushing those bounds. It’s never been easier to parse and analyze a truly vast amount of structured data.
Yet, the way we consume and interpret unstructured data has remained unchanged. Besides Command+F and Sparknotes, there really haven’t been any tools that have meaningfully increased our natural bandwidth for processing documents, images, and videos. There is no “Excel” for the rapidly growing pool of unstructured information, so to speak.
The opportunity here is building one.
Market Leadership
Becoming a market leader is a two-step process: you’ve got to gain a lot of market share from incumbents and you’ve got to defend it from competitors.
Let’s look at how Hebbia might fare on both.
Gaining Market Share
To gain significant market share, you have to be much better than what people are currently using in some way. If you’re only slightly better, people won’t bother moving their data, workflows, and habits. This is why venture capitalists often say you’ve got to “10x” something about the user experience.
So what are people currently using to search through large amounts of unstructured data?
Primarily, it’s Command+F and Adderall.
While basic semantic search systems have been around for a while (i.e. when you search for products on Amazon), nothing today is precise or granular enough to power a standalone search product. The major problem is lossy transformation: when you use an embedding model to convert documents into a vectors of numbers (the foundation of all semantic search systems), nuance in the language can be lost. Traditional semantic search systems are unable to pinpoint the exact sentences or paragraphs that best answer your question, especially when the corpus is complex and domain-specific. They can tell you which documents are similar to your question, but they can’t tell you why.
When you’re searching for products or Reddit posts, this is fine - there’s a higher acceptable margin of error. But for high-stakes tasks requiring any amount of precision, it’s just not good enough. As a result, everyone still opens up a bunch of tabs and keyword-searches their way through them.
Hebbia’s product achieves the precision of Command+F with the robustness of semantic search by solving fundamental technical problems with both:
Compared to traditional semantic search systems, Hebbia is more precise. From a user perspective, Hebbia’s proprietary indexing system offers not just the most relevant documents, but also the most relevant sentences and paragraphs within those documents. This enables them to offer citations, a critical feature for any researcher. From a technical perspective, Hebbia’s system outperforms current state-of-the-art information retrieval systems by 57% (as measured by mean reciprocal rank on standard datasets).
Compared to Command+F, Hebbia is faster and more robust. By leveraging the intent of your query, Hebbia prevents you from worrying about synonyms or phrasing - you’ll find resources you might otherwise have missed due to semantics. By ordering results using artificial intelligence, Hebbia prevents you from going through every hit like you’d have to normally.
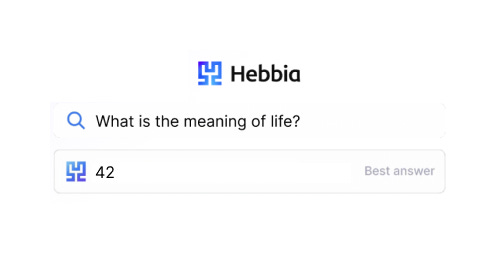
Beyond solving important technical challenges, Hebbia has also built out all the features needed for a standalone search product. These include:
Centralized, MIME type-agnostic search. Hebbia allows you to search through PDFs, Word documents, transcripts, emails, and Excel files all in one go. Support for hybrid search allows you to filter results using keywords. This is much better than opening a bunch of windows and searching through files one at a time.
End-to-end encryption. Hebbia’s search index is end-to-end encrypted, giving it protection both at rest and in-transit. This is critical given the sensitive information their clients are often searching through.
Collaborative tools. Hebbia has all the collaborative features you’d expect from a 21st century SaaS tool. A strict role-based access control system ensures sensitive information is only ever seen/searchable by the right people.
By blending the unique strengths of both Command+F and neural search, Hebbia provides an experience thats significantly faster and better for its users. In this, the user experience is 10x’ed: going from Command+F to Hebbia is like going from Robert’s Tabula to Command+F in the first place.
As a result, Hebbia has gone from 0 to 90% market penetration within top private equity firms in less than a year. They’re now rapidly expanding from that initial userbase.
Talk about rapidly gaining market share.
Defending Market Share
To defend your market share, you need moats to stave off copycats. Let’s have a look at some of Hebbia’s.
Technical moats. Hebbia isn’t just another CRUD app - it’s a real technical feat. Important innovations include:
Near-lossless embedding transformation, which allows their index to scale naturally with exponential increases in document volume.
Proprietary encoders and indexers, which allow Hebbia to outperform state-of-the-art information retrieval systems by 57% (measured on mean reciprocal rank).
MIME type-agnostic parsing, which allows them to read, index, and query many different file types in one cohesive system.
Layout analysis and tabular data extraction, which gives their engine more context to offer better suggestions.
Hybrid search, which allows users to search with additional filters (i.e. only PDFs and must include this keyword).
End-to-end encryption, which keeps their users’ data safe.
Your average startup doesn’t have this degree of technical depth.
High switching costs. By design, Hebbia will naturally become the system of record for all unstructured information related to research and business intelligence. As customers continue to upload files, share findings, and make decisions on Hebbia, moving away becomes more difficult.
Competitive Landscape
Hebbia has many unique strengths, but they’re far from the only ones working in the world of AI-enhanced search.
Now that we have an understanding of Hebbia’s product and approach, let’s have a look at their potential competitors. Note our use of “potential” - while we couldn’t find any direct competitors selling the same product to the same market, a future competitor would likely spawn out of one of the following categories.
Semantic Search APIs
If you look up something along the lines of “AI-powered search,” this is the first batch of startups you’ll come across. These companies offer APIs to help other companies build semantic search into their own products - think a Reddit, Discord, or Slack looking to upgrade search on their platforms. Some notable players include:
Vectara - an LLM-powered search-as-a-service API that offers developers a complete ML search pipeline. They recently raised a $20 million seed round from Sparq Capital, Transform VC, GTM Capital, and a number of other investors.
Jina.ai - behind Jina, an open-source multi-modal neural search framework. Their managed offering embeds everything from text to videos before handling search for you. They recently raised a $30 million Series A led by Canaan Partners.
Deepset.ai - behind Haystack, an open-source developer framework for building NLP features like document extraction and similarity. They have a managed offering and recently raised a $14 million Series A led by GV.
While these companies aren’t competitors since they don’t sell to end-users directly, it’s worth asking how they might be leveraged to build a competing end-to-end search product like Hebbia.
While these services provide a helpful starting point, they’re primarily meant for businesses who don’t rely on search as their main product. The gulf between a demo built with one of these APIs and a usable, standalone search tool is vast: depending on the platform you pick, you’d still have to add encryption, parsers for different file types, model tuning, collaboration features, citations, and summaries yourself. Comparing a framework to a finished product like Hebbia would be like comparing SQL to Excel.
Managed Search
The next bucket of companies are ones that do offer an end-to-end search experience. Like Hebbia, they also sell to businesses and are utilized directly by end-users. Unlike Hebbia, they all focus on different niches and use-cases: “search” is a general enough technology that startups have to niche-down in order to find specific use-cases people are willing to pay for. Some players in this category include:
Glean - a tool that unifies search across all of a company’s SaaS apps. They leverage semantic search models tuned to individual organizations to deliver personalized query responses. They recently raised $100 million at a $1 billion valuation led by Sequoia.
Relevance AI - a tool that leverages semantic search to help marketers understand and tag customer feedback at scale. They recently raised $3 million from Insight Partners.
While these and many other products each leverage AI-powered search, they all appear to target fundamentally different markets and use-cases (as expressed in their feature sets, integrations, and marketing). Glean, for instance, has strong traction among other technology companies and as a result offers connectors for all the popular SaaS apps they use. Hebbia, in contrast, has the strongest focus on and traction among professional services firms.
Foundation Models
It’s hard to discuss anything NLP-related without acknowledging the broad, disruptive power of foundation models like GPT-3. To set the stage, some major players in this camp include:
OpenAI - the developer of text-generating systems like GPT-3/ChatGPT and image-generating systems like DALL·E 2. They recently received a multi-billion dollar investment from Microsoft.
Cohere - the developer of text-generating systems, embedding systems, and natural language classification systems available to developers via API. They recently raised $125 million from Tiger Global.
Anthropic - the developer of text-generating systems like Claude. They previously raised $580 million from investing great Sam Bankman-Fried.
It’s worth asking how generative AI systems might be used to compete against tools like Hebbia. While anything is possible, this isn’t likely in the foreseeable future. The key is in the word “generative”: these models are really good at writing text, but not so much at reading and interpreting it like search engines. Language models have no inherent source of truth and are susceptible to generating probable-sounding nonsense. Trying to inform them by cramming the entire search corpus into context (the prompt) or training data is difficult due to input/output length limitations and training costs.
As a result, it’s tough to see a world where search engines rely solely on large language models. Rather, it’s more likely they’re used alongside a source of truth like Hebbia. George wrote an excellent piece here explaining why, but we also go into more depth below.
Execution
Hebbia has a highly ambitious, highly technical product vision. To execute, they’ll need a highly ambitious and highly technical team.
After looking through the LinkedIn profiles of everyone in the company, we can confirm this is the case. Hebbia is currently under 20 full-time employees and skews younger, highly ambitious, and deeply technical. Most hail from top tech companies, elite startups, and strong engineering programs. We discovered we had already known several personally from Stanford.
Consider the roster for yourself:
David Morse - Chief Customer Officer
David was previously VP Automotive and Robotics at Scale AI, Director of Customer Success at Dropbox, and a strategy consulting manager at Accenture. He holds an MBA from Northwestern and a B.A. from Dartmouth.
Swetha Revanur - Engineering Manager
Swetha was previously the first machine learning engineer at Brex. Before that, she was a machine learning engineer at Amazon. She holds a B.S. in CS from Stanford, where she is on leave from her Masters in CS. Usman has taken a class with her before and can attest to how great she is.
Lainie Yallen - Product Manager
Lainie was previously Co-founder and Head of Growth at TriplePlay, a startup acquired by Roblox. Before that, she was a consultant at BCG. She holds a BCom in Entrepreneurship and Finance from McGill.
Jayen Ram - Software Engineer
Jayen was previously a Team Lead at Dandy. Before that, he was a data scientist on the Biden campaign and a software engineer at Facebook. He holds a B.S. in CS from Stanford, and was in Sigma Phi Epsilon with Usman. He’s amazing at basketball.
Ammar Husain - Software Engineer
Ammar was previously a software engineer at Meta, a machine learning engineer at Snap, and a machine learning engineer at Salesforce. He holds a B.S. in CS from Purdue.
Lucas Haarmann - Software Engineer
Lucas was previously a data scientist at PIMCO, an investment firm managing roughly 2 trillion dollars. He holds a B.S. in ME from Stanford, and was Usman’s pset partner at one point. We can vouch for his problem-solving ability.
Cooper Raterink - Machine Learning Engineer
Cooper was previously a machine learning engineer at Cohere, the OpenAI competitor. He holds a B.S. in EE from UT Austin and an M.S. in CS from Stanford.
Ryan McCaffrey - Software Engineer
Ryan was previously a software engineer at Robinhood and a technology associate at Bridgewater. He holds a B.S. in engineering from Princeton.
Ethan Hart - Business Development Manager
Ethan previously worked in investment banking at Credit Suisse. Before that, he was an analyst at EJF Capital and Village Capital, a hedge fund and venture capital firm. He holds a B.S. in Finance from BYU.
Nicholas Clift - Head of Customer Operations
Nicholas was previously Director of Client Solutions at Alloy and an Engagement Manager (consultant) with McKinsey. He holds a B.S. and M.S. in EE from the University of Michigan.
Vedran Hadziosmanovic - Software Engineer
Vedran was previously a machine learning engineer at Microsoft and Predata, where he developed NLP models for unstructured web data. He holds a B.A. in CS from UC Berkeley.
Max Martin - Account Executive
Max was previously a founding AE at Balance. Before that, he drove sales at Alice and Spring Health. He holds a B.A. in History from Yale University, where he led their varsity squash team to National championships.
Andoni Tsougarakis - Business Operations
Andoni was previously an investment banking associate at MUFG, Japan’s largest bank. He holds a B.A. from Cornell University.
As you can see, the team contains a strong mix of people with AI/ML experience and people with consulting/investing backgrounds.
This is an excellent combination in any case, but it’s especially fitting here given the product Hebbia is building and the customers they’re selling to.
The Long Term
In the short term, Hebbia has many opportunities to expand. They can expand into new markets, add support for more file types, and build features to cement their role as the system of record for firms employing knowledge workers.
In the long term, though, things get much more interesting.
Today, everybody’s focused on generative AI - systems like ChatGPT and DALL·E 2, which can write or paint for you. With tools like these, the marginal cost of producing content approaches zero. Soon, the world will have more emails, blog posts, and briefings than it’ll know what to do with.
But that’s exactly the problem.
For many years now, the bottleneck in our relationship with knowledge hasn’t been a lack of quality content - the internet made sure of that.
Rather, it’s been our individual bandwidths for information consumption - nobody has enough time to read and understand all the content we do have. Giving people more to consume doesn’t fundamentally change anything - as we described at the start, there’s got to be a balance between our ability to create information and our ability to consume it.
As tools like ChatGPT make content creation orders of magnitude easier, we’ll all need systems that make reading and analyzing it that much easier as well. As a result, semantic search systems like Hebbia are the natural counterparts to generative systems like ChatGPT.
As ChatGPT writes, Hebbia reads.
Conclusion
If you were to travel back in time to show Robert Grosseteste and the rest of England a laptop running Hebbia, you’d probably get burned at the stake for witchcraft or something.
They didn’t have computers back then.
Once you’d taken the time to show them how things worked, however, Robert would probably thank you for making his and his students’ lives a lot easier: Tabula was fine in concept, but it was kind of unusable for anyone besides Robert due to his crappy handwriting.
Robert’s been gone for many years, but there are still many like him today toiling away at law firms, consulting firms, and investment firms - they’re just using Command+F instead of hacky hand-written indices.
It’s about time they all got an upgrade. Hebbia’s hiring: https://boards.greenhouse.io/hebbia.
Thanks for reading! In case you missed our previous pieces, check them out here:
And to make sure you don’t miss any future ones, be sure to subscribe here:
Finally, if you’re a founder or investor with a company you think we should cover please reach out to us at ericzhou@stanford.edu and uhanif@stanford.edu - we’d love to hear about it :)
This is awesome, Thank you!!
This is my introduction to your work/substack. If I may, what purpose for joining a startup does your coverage assumes please?